The Art of Forgetting: How AI Models Are Learning to Let Go
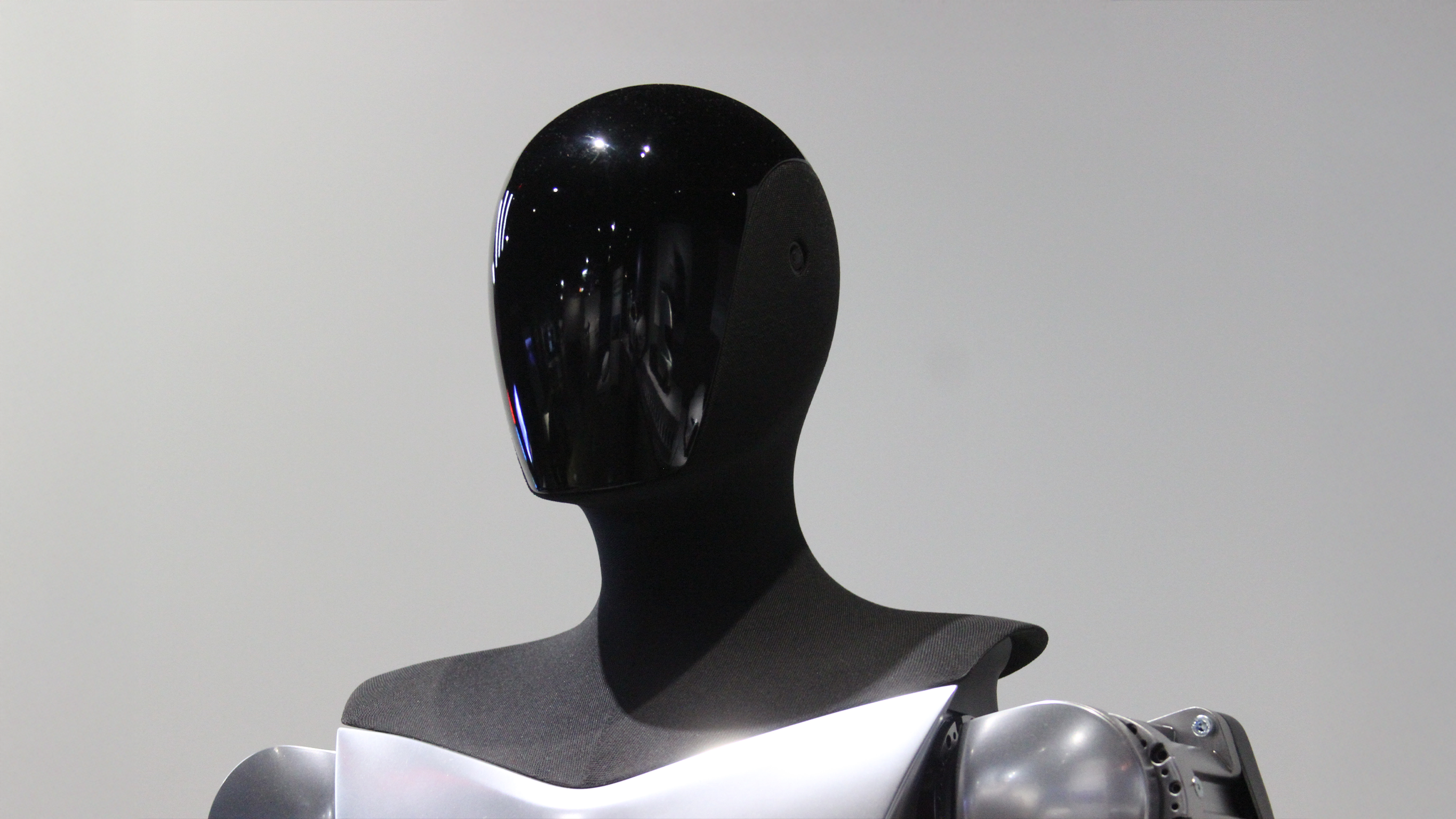
AI is evolving to do something truly human—forget what it doesn’t need. Researchers are teaching machines to selectively erase data, boosting privacy, efficiency, and precision.
The Challenge of Overloaded AI Models
Large-scale AI models have transformed industries, from healthcare diagnostics to autonomous vehicles. Their generalist capabilities allow these systems to handle a vast range of tasks, often with astonishing precision. However, this versatility comes at a cost: increased computational complexity, higher energy consumption, and ethical challenges around privacy and efficiency.
Imagine an autonomous driving system that’s also trying to identify couches, burgers, and pet giraffes. Helpful? Not so much. These systems thrive when focused on what’s essential—cars, pedestrians, and traffic signs. Retaining irrelevant data wastes energy, eats up resources, and lowers overall accuracy. It’s like trying to run a marathon with a backpack full of rocks.
This is where researchers at the Tokyo University of Science (TUS) step in. They’ve pioneered a breakthrough: enabling AI systems to selectively forget unnecessary or sensitive data. Dubbed “machine unlearning”, this innovation not only streamlines AI models but also helps address growing concerns around privacy and compliance.
Black-Box Forgetting: A New Frontier
AI models can generally be categorized into white-box and black-box systems. White-box systems provide visibility into their inner workings—making it easier to modify or remove data. But the majority of today’s AI systems are black boxes, where internal architecture is locked away like a secret recipe. Ethical and commercial restrictions keep the internal mechanisms off-limits.
This creates a real problem when we need these systems to “forget” specific information, especially with compliance laws (like GDPR’s Right to be Forgotten) requiring sensitive data removal. Enter the Tokyo University of Science team, who turned to derivative-free optimization—a clever workaround that doesn’t require peeking inside the model’s brain.
At the heart of their approach is an optimization strategy known as Covariance Matrix Adaptation Evolution Strategy (CMA-ES). Presented at the Neural Information Processing Systems (NeurIPS) conference, the method involves tweaking input prompts until the model “forgets” certain classes of data. Tested on the vision-language model CLIP, this strategy reduced targeted classes by a striking 40% without retraining or altering the model’s core.
Why Machine Unlearning Matters
-
Efficiency & Sustainability: AI models are data-hungry beasts. Machine unlearning trims the fat, reducing computational demands, speeding up performance, and cutting energy use. Lighter, faster models can run on smaller devices—unlocking new possibilities in industries where size and power constraints matter.
-
Privacy & Compliance: Large datasets often contain sensitive or outdated information. Machine unlearning gives enterprises a practical way to comply with privacy laws like GDPR without the expensive, energy-intensive process of retraining entire models.
-
Content Moderation: Ever seen an AI generate something it really shouldn’t have? Machine unlearning helps prevent models from creating harmful or inappropriate content by removing unwanted visual categories.
-
Sharper Focus: Models that shed irrelevant data can zero in on what’s truly important for the task at hand. Whether it’s autonomous vehicles or medical diagnostics, precision is everything.
Building Smarter, More Responsible AI
The tension between innovation and responsibility in AI is growing. Machine unlearning offers a practical solution: models that are not only smarter and faster but also more ethical and sustainable.
The Tokyo University of Science’s approach to black-box forgetting isn’t just a technical milestone—it’s a reminder that less can be more. By teaching machines to forget, we’re helping them focus, adapt, and operate with fewer risks and resources. At Medialake, we believe innovations like these will define the future of intelligent systems.
It’s not just about what AI can learn. It’s also about what it can let go.
Want to stay ahead in AI and enterprise intelligence? Explore how Medialake helps brands unlock insights, optimize performance, and govern data effectively across their marketing ecosystems.